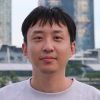
Dr Weiming Huang
- Position: Lecturer in Urban Data Science
- Areas of expertise: Geographical Information Science; Urban Analytics; Machine Learning; Knowledge Graph; Data Mining
- Email: W.Huang@leeds.ac.uk
- Location: 10.123 Irene Manton Building
- Website: Twitter | Googlescholar | Researchgate | ORCID
Profile
I am a Lecturer in Urban Data Science at the School of Geography and a member of the Institute for Spatial Data Science. Before joining Leeds in 2024, I had a blended background in Geographical Information Science (GIScience) and Computer Science. I obtained my PhD in GIScience from Lund University, Sweden, and was a Wallenberg Postdoctoral Fellow at Nanyang Technological University, Singapore, and Lund University, primarily in a Computer Science environment.
My research centres on the technical aspects of GIScience and their applications in both urban and natural environments. Specifically, I focus on organising and analysing various geospatial data modalities, such as points of interest, street view images, and human trajectories, by developing or utilising state-of-the-art techniques in machine learning and semantic web technologies (knowledge graphs).
With these research lines, I have published more than 40 articles in leading GIScience journals and top-tier Computer Science conferences. I have also served as a guest editor for IJGIS, TGIS, JAG, and the Semantic Web journal, and have acted as a programme committee member for a number of prestigious machine learning/data mining conferences, including NeurIPS, ICML, and ICLR.
Major Grants & Awards
• Waldo Tobler Young Researcher Award from Austrian Academy of Sciences, 2022
• EuroSDR Award for the Best PhD Thesis Related to Geoinformation Science, 2021
• Wallenberg Postdoctoral Fellowship at Nanyang Technological University and Lund University, 2020
Research interests
My research leverages both top-down, theory-informed approaches and bottom-up, data-driven methods (the two pathways of GeoAI), to deepen our understanding of the complex urban and natural environments, and finally inform decision-marking towards sustainable development. The full list of my publications can be found here. My research can be broadly grouped into three main research themes:
Spatial data mining for urban analytics: The growing proliferation of urban sensing data sources calls for effective data mining methods to extract useful insights from vast amounts of data. In this context, my research seeks to learn general-purpose and effective representations (vector embeddings) from a variety of urban sensing data. These representations then serve as effective features to enhance the performance of various urban analyses. We have developed novel representation learning methods using a wide array of data modalities, e.g., points of interest (POIs) (Huang et al. 2023), OpenStreetMap (Li et al. 2023), street view images (Zhou et al. 2024), remote sensing data (Bai et al. 2023), and human trajectories (Zhang et al. 2024). These studies have demonstrated the effectiveness of representation learning in urban analytics, such as inferring functional land use, population density, traffic speed, house prices, individual next locations, and crime rates.
Geospatial foundation model: The recent surge of foundation models, e.g., GPT-family models, has sparked great interests in GeoAI. In this vein, we have first thoroughly evaluated how general-purpose foundation models perform in geospatial and urban tasks, showing that these models often fall short in spatial and urban contexts (Mai et al. 2024). This underscores the need for foundation models specifically tailored to geospatial challenges. To this end, we have accomplished the development of a preliminary version of City Foundation Model (CityFM), in which representations of various entities from OpenStreetMap within a city are jointly learned, leading to improved performance across a range of urban analyses (Balsebre et al. 2024). In a parallel line of research, we have been extensively studying, utilising and further developing vision-language models for geospatial tasks. We proposed the UrbanCLIP framework to enable the pretrained vision-language model CLIP to effectively infer fine-grained urban land use in a zero-shot manner, i.e. with no additional model training or labelled samples (Huang et al. 2024). Currently, I am actively developing foundation models for urban analyses.
Geospatial knowledge graph: Geospatial data integration and the formalisation of geospatial knowledge have been long-standing challenges in GIScience, and are critical for various geospatial analyses. My research explores the use of knowledge graphs for two main purposes: 1) integrating multi-source geospatial data, and 2) formalising geovisualisation knowledge to enhance its interpretation, transfer, and reuse. To this end, we have developed several knowledge bases for geovisualisation, e.g. for heritage building and natural reserve analyses (Huang et al. 2017, 2020). Our benchmarking work has been used by European Spatial Data Research (EuroSDR).
Student education
I presently teach several subjects within the Data Science sector.
Research groups and institutes
- Institute for Spatial Data Science