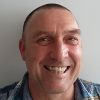
Professor Lex Comber
- Position: Chair in Spatial Data Analytics
- Areas of expertise: Spatial analysis; GeoComputation; Spatial planning; Spatio-temporal modelling, Spatial inequalities; Data bias; Critical sata science; Location-allocation; Land cover; Land use; Scale effects
- Email: A.Comber@leeds.ac.uk
- Phone: +44(0)113 343 9225
- Location: 10.118 Irene Manton Building
- Website: Twitter | LinkedIn | Googlescholar | Researchgate | ORCID
Profile
Alexis Comber, Lex, is Professor of Spatial Data Analytics at Leeds Institute for Data Analytics (LIDA) the University of Leeds. He worked previously at the University of Leicester where he held a chair in Geographical Information Science. His first degree was in Plant and Crop Science at the University of Nottingham and he completed a PhD in Computer Science at the Macaulay Institute, Aberdeen (now the James Hutton Institute) and the University of Aberdeen developing expert systems for land cover monitoring. This brought him into the world of spatial data, spatial analysis, and mapping. Lex’s interests span many different application areas including land cover / land use, demographics, public health, agriculture, bio-energy and accessibility, all of which require multi-disciplinary approaches. His research draws from geocomputation, mathematics, statistics and computer science and he has extended techniques in operations research / location-allocation (what to put where), graph theory (cluster detection in networks), heuristic searches (how to move intelligently through highly dimensional big data), remote sensing (novel approaches for classification), handling divergent data semantics (uncertainty handling, ontologies, text mining) and spatial statistics (quantifying spatial and temporal process heterogeneity).
With the wider use of spatial data in other disciplines (actually all data are spatial – they are collected some-where!), Lex’s collaborations are increasingly with researchers in non-geographical domains. Recent examples include mental health, consumer analytics, market segment dynamics, bio-informatics and spatial transcriptomics.
He has co-authored (with Chris Brunsdon) the first ‘how to book’ for spatial analyses and mapping in R the open source statistical software, An Introduction to R for Spatial Analysis and Mapping, now in its second edition (https://uk.sagepub.com/en-gb/eur/an-introduction-to-r-for-spatial-analysis-and-mapping/book258267). The follow on to this is Geographical Data Science and Spatial Data Analysis: and Introduction in R, (ISBN: 978-1526449351, was published in 2021 (https://us.sagepub.com/en-us/nam/geographical-data-science-and-spatial-data-analysis/book260671). Outside of academic work and in no particular order, Lex enjoys his vegetable garden, walking the dog and playing pinball (he is the proud owner of a 1981 Bally Eight Ball Deluxe).
Responsibilities
- Programme Leader for the Data and Society CDT (CDAS - https://datacdt.org)
Research interests
My research in Spatial Data Analytics develops methods that integrate and analyse the high volumes of spatial data that are being ubiquitously generated by our digital transactions, in order to uncover hidden patterns / correlations. It provides spatial insight and supports spatial planning by linking across both social and environmental dimensions, but always through a data critical eye (ie with a critica data science approach). The context for this work is the limitless demand for and capture of spatial data: from smartphone apps, social networks and more traditional activities related to planning, human rights, environmental justice, public health, climate change, agriculture, land use, etc. This also extends concepts of 'crowd sourcing' into the 'citizen sensor', supporting community empowerment, resilience and adaptability. Some of the key challenges in this work are understanding the uncertainties associated with linking data describing different processes but captured over different spatial supports (scales), and dealing with issues of data bias and representativeness.
My current research is focused on 2 main areas: 1) Developing methods to support decision making at different scales, that are able to quantify cross scale trade-offs with any decision. These are being applied to spatial planning and land use in both urban / peri-urban and rural contexts, and to sample designs that support multiple decision scales (e.g. from household to community to settlement, and from field to farm to catchment). 2) Developing methods to quantify process spatial and spatial heterogeneity (how, where and when processes vary). These are being used to examine how the drivers of processes vary spatially and temporally and are being applied to understand neighbourhood change (such as gentrification), spatial inequalities in health outcomes and the emergence of house price bubbles. An example of how these research themes combine is the development of a tools to support spatial decisions and spatial planning given a) existing spatial patterns and distributions, b) different underlying social and environmental gradients, c) the value placed on different activities and process, and d) different scales of decision making.
Current PhD Students
- Arif Rohman (with Gordon Mitchell), Natural flood management in tropical climates (started Sept 2017)
- Yiyu Wang (with Jiaqi Ge) A simulation model of pedestrian flow using Bayesian Nash equilibrium in a multi-agent system (started October 2020)
- Lisma Safitri (with Marcelo Galdos & Andy Challinor) Water use efficiency monitoring using artificial intelligence (started October 2021)
- Raidah Hanifah (with Nick Malleson & Vikki Houlden) Spatial temporal analysis of tourism travel pattern from social media data (started October 2021)
- Eleftherios Zormpas (with Simon Cockell, Newcastle University) Mapping the transcriptome: using geocomputational methods to unlock the potential of spatial transcriptomics data (started January 2022)
- Holly Moore (with Thomas Reynolds, University of Edinburgh) Characterising Cyclone Damage Using Earth Observation Data (started October 2022)
Teaching
I convene the following modules in 2023/24:
- Big Data and Consumer Analytics (GEOG5917)
- GeoComputation and Spatial Analysis (GEOG3195)
- Digital Geographies (GEOG1400)
Recent Publications (last 3 years)
-
Comber A, Harris P and Brunsdon C (2023). Multiscale spatially varying coefficient modelling using a Geographical Gaussian Process GAM. International Journal of Geographical Information Science, https://doi.org/10.1080/13658816.2023.2270285
-
Comber A and Tsutsumida N (2023) Geographically weighted accuracy for hard and soft land cover classifications: 5 approaches with coded illustrations. International Journal of Remote Sensing, http://dx.doi.org/10.1080/01431161.2023.2264503
-
Gray J, Buckner L and Comber A (2023). Predicting Gentrification in England: A Data Primitive Approach. Urban Science, 7(2): 64; https://doi.org/10.3390/urbansci7020064
-
Wang Y, Ge J and Comber A (in press). An Agent-Based Simulation Model of Pedestrian Evacuation Based on Bayesian Nash Equilibrium. Paper accepted for publication by Journal of Artificial Societies and Social Simulation (April 2023), https://arxiv.org/abs/2211.14260
-
Gray J, Buckner L and Comber A (2023). Identifying Neighbourhood Level Change Using a Data Primitive Approach: Gentrification in South Yorkshire. Applied Spatial Analysis and Policy, 16(2): 897-921 10.1007/s12061-023-09509-y
-
Kieu M, Comber A, Wanjau E, Bratkova K, Hang NTT, Thanh BQ, Phe HH, and Malleson N (2023). Factors affecting perceptions in transport - A deep dive into the motorbike ban in Hanoi, Vietnam, Case Studies on Transport Policy, 11: 100958, https://doi.org/10.1016/j.cstp.2023.100958
-
Comber A, Brunsdon C, Charlton M, Dong G, Harris R, Lu B, Lü Y, Murakami D, Nakaya T, Wang Y and Harris P (2023). A route map for successful applications of Geographically Weighted Regression. Geographical Analysis, 55(1):155-178, https://doi.org/10.1111/gean.12316
-
Lu B, Hu Y, Yang D, Liu Y, Liao L, Yin Z, Xia T, Dong Z, Harris P, Bundsdon C, Comber L and Dong G (2022). GWmodelS: a software for geographically weighted models. SofwareX, 21: 101291, https://doi.org/10.1016/j.softx.2022.101291
-
Comber A, Harris P, and Brunsdon C (2022). A Rejoinder to the Commentaries on “A Route Map for Successful Applications of Geographically Weighted Regression” by Comber et al. (2022). Geographical Analysis, 55(1):198-202, https://doi.org/10.1111/gean.12352
-
Cui N, Malleson N, Houlden V and Comber A (2022). The impact of the COVID-19 pandemic on urban green space use. Urban Forestry & Urban Greening, 74: 127677, https://doi.org/10.1016/j.ufug.2022.127677
-
Comber A, Callaghan M, Harris P, Lu B, Malleson N and Brunsdon C (2022). gwverse: a template for a new generic Geographically Weighted R package. Geographical Analysis, 54: 685-709, https://doi.org/10.1111/gean.12337
-
Lu B, Hu Y, Murakami D, Brunsdon C, Comber A, Charlton M and Harris P (2022). High-performance solutions of geographically weighted regression in R. Geo-spatial Information Science, https://doi.org/10.1080/10095020.2022.2064244
-
Song Y, Wang Y, Jin L, Shi W, Aryal J and Comber A (2022). Quantitative contribution of the Grain for Green project to vegetation greening and its spatiotemporal variation across the Chinese Loess Plateau. Land Degradation & Development, 33(11): 1878-1891 https://doi.org/10.1002/ldr.4269
-
Comber A and Harris P (2022). The importance of scale and the MAUP for robust Ecosystem Service evaluations and landscape decisions. Land 11(3): 399 https://doi.org/10.3390/land11030399
-
Yang Y, Beecham R, Heppenstall A, Turner A and Comber A (2021). Understanding the impacts of public transit disruptions on bikeshare schemes and cycling behaviours using spatiotemporal and graph-based analysis: A case study of four London Tube strikes. Journal of Transport Geography. https://doi.org/10.1016/j.jtrangeo.2021.103255
-
Gadd SC, Comber A, Tennant P, Gilthorpe MS and Heppenstall AJ (2021). The utility of multilevel models for continuous-time feature selection of spatio-temporal networks. Computers, Environment and Urban Systems, https://doi.org/10.1016/j.compenvurbsys.2021.101728
-
Cui N, Malleson N, Houlden V and Comber A (2021). Using VGI and social media data to understand urban green space: A narrative literature review. International Journal of Geo-Information, 10: 425, https://doi.org/10.3390/ijgi10070425
-
Gray J, Buckner L, Comber A (2021). Extending Geodemographics Using Data Primitives: A Review and a Methodological Proposal. ISPRS International Journal of Geo-Information, 10(6):386, https://doi.org/10.3390/ijgi10060386
-
Yi-Min CC, Carver S, Comber A (2021). An exploratory analysis of the formal and informal landscape aesthetics evaluations: a case study from Wales. Land, 10(2): 192 https://doi.org/10.3390/land10020192
-
Gosal AS, Giannichi ML, Beckmann M, Comber A, Massenberg JR, Palliwoda J, Roddis P, Schägner JP, Wilson J and Ziv G (2021). Do drivers of nature visitation vary spatially? The importance of context for predicting visitation of nature areas in Europe and North America. Paper accepted by Science of the Total Environment https://doi.org/10.1016/j.scitotenv.2021.145190
-
Gadd SG, Comber A, Gilthorpe MS, Suchak K and Heppenstall A (2021). Simplifying the interpretation of continuous time models for spatio-temporal networks. of Geographical Systems, 24(2):171-198, https://doi.org/10.1007/s10109-020-00345-z
-
Fu Y, Deng J, Wang H, Comber A, Yang W, Wu W, You S, Lin Y and Wang K (2021). A new satellite-derived dataset for marine aquaculture in the China’s coastal region. Earth System Science DataEarth System Science Data, 13(5): 1829-1842, https://doi.org/10.5194/essd-2020-122
-
Brunsdon C and Comber A* (2020). Big Issues for Big Data: challenges for critical spatial data analytics. Journal of Spatial Information Science, 21: 89–98, https://doi.org/10.5311/JOSIS.2020.21.625
-
Chien Y-MC, Carver S and Comber A (2020). Using geographically weighted models to explore how crowdsourced landscape perceptions relate to landscape physical characteristics. Landscape and Urban Planning, https://doi.org/10.1016/j.landurbplan.2020.103904.
-
Brunsdon C and Comber A* (2021). Opening practice: supporting Reproducibility and Critical spatial data science. Journal of Geographical Systems, 23:477-496, https://doi.org/10.1007/s10109-020-00334-2
-
Harris P, Lanfranco B, Lu B and Comber A (2020). Influence of geographical effects on hedonic pricing models for grass-fed cattle in Uruguay. Agriculture, 10, 299, https://doi.org/10.3390/agriculture10070299
-
Li, Z., White, J.C., Wulder, M.A., Hermosilla, T., Davidson, A.M., Comber, A.J. (2020). Land cover harmonization using Latent Dirichlet Allocation. International Journal of Geographical Information Science, https://doi.org/10.1080/13658816.2020.1796131
-
Bell MJ and Comber A (2020). Smarter Farming: New Approaches for Improved Monitoring, Measurement and Management of Agricultural Production and Farming Systems. Frontiers in Sustainable Food Systems, https://doi.org/10.3389/fsufs.2020.00121
-
Yang Y, Heppenstall A, Turner A, Comber A (2020). Using graph structural information about flows to enhance short-term demand prediction in bike-sharing systems. Computers, Environment and Urban Systems, https://doi.org/10.1016/j.compenvurbsys.2020.101521
-
Westerholt R, Mocnik F-B and Comber A (2020). A place for place – Modelling and analysing platial representations. Transactions in GIS, https://doi.org/10.1111/tgis.12647
-
Belete M, Deng J, Teshome M, Wang K, Woldetsadik M, Zhu E, Comber A, Gudo A and Abubakar GA (2020). Partitioning the Impacts of Land Use/Land Cover Change and Climate Variability on Water Supply over the Source Region of Blue Nile Basin. Land Degradation and Development, https://doi.org/10.1002/ldr.3589
-
Beecham R, Williams N and Comber A (2020). Regionally-structured explanations behind area-level populism: An update to recent ecological analyses. PLoS ONE 15(3): e0229974. https://doi.org/10.1371/journal.pone.0229974
-
Comber A, Chi K, Huy MQ, Nguyen Q, Lu B, Phe HH and Harris P (2020). Distance metric choice can both reduce and induce collinearity in geographically weighted regression. Environment and Planning B, 47(3): 489–507 https://doi.org/10.1177/2399808318784017
-
Ren Y, Lü Y, Fu B, Comber A, Li T, Hu J (2020). Driving factors of land change in China’s Loess Plateau: quantification using Geographically Weighted Regression and management implications. Remote Sensing 12(3),453; https://doi.org/10.3390/rs12030453
-
Zeng W and Comber A (2020). Using household counts as ancillary information for areal interpolation of population: comparing formal and informal, online data sources. Computers, Environment and Urban Systems, 20 https://doi.org/10.1016/j.compenvurbsys.2019.101440
<h4>Research projects</h4> <p>Some research projects I'm currently working on, or have worked on, will be listed below. Our list of all <a href="https://environment.leeds.ac.uk/dir/research-projects">research projects</a> allows you to view and search the full list of projects in the faculty.</p>
- Integrated Analysis of Social Media and Hedonic House Prices for Neighbourhood Change (INTEGRATE)
- Modelling and managing critical zone relationships between soil, water and ecosystem processes across the Loess Plateau
- Real-time predictions of pesticide run-off risk which: multi-scale visualisations of water quality risks and costs
Research groups and institutes
- Institute for Spatial Data Science