Modelling the future of cities
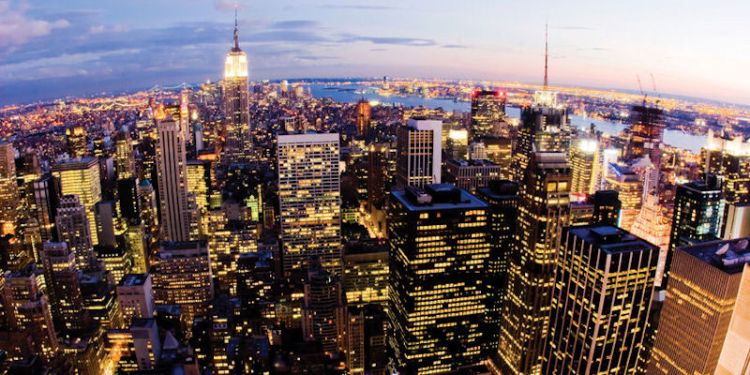
Predicting how cities will develop in the future, and how best to plan for growth, is being made easier for policymakers through advanced modelling techniques being developed by Professor Alison Heppenstall and colleagues, working in the field of urban analytics, in the School of Geography.
Alison’s research focuses on building computational models to understand how people use cities and the processes that drive cities from an individual’s perspective. She is interested in creating individual-based models of how people move around and behave in the city space.
There has been a big shift in recent years in the way we think about cities through the advent of ‘Big Data’. The digital economy provides a wealth of new data on how people behave in cities. Data from phones, public transport, pollution monitors and supermarket transactions are just a few of the information streams about people’s use of the urban environment that can now feed into research.
“Previously, cities were thought of as big homogeneous units as we didn’t have the data” explains Alison. “Now ‘Big Data’ coming in means we can start building pictures from that and asking questions like ‘who are these people and where are they going next?’”
Previously, cities were thought of as big homogeneous units as we didn’t have the data. Now ‘big data’ means we can start building detailed pictures...
The aim is to understand how the consequences of individuals behaviour and decisions play out over time. This will give local authorities, health care providers and essential services like the police, the information they need to plan for different future scenarios.
“Cities are social systems that are driven by thousands of individual decisions,” explains Alison. “Cities work as individuals networking together. These networks operate over different scales to shape and drive cities.”
Her expertise in understanding these urban networks during the 2020 COVID-19 pandemic has been particularly important. Her team are building models to answer vital long-term questions about how best cities can transition out of lockdown and how to get the economy moving again.
Agent-based and microsimulation modelling
To understand cities from an individual’s perspective, modelling techniques that simulate individuals and their decisions are needed. Agent-based modelling and microsimulation are two techniques that Alison and her research team are using and refining to understand cities at a microscale.
By looking for trends and signals in the data over space and time, and piecing these together using machine learning, the researchers can build better models of how individuals (agents) move in cities – this is agent-based modelling. Models can then be used to make better forecasting predictions for different facets of city life. This has included working closely with several police forces to model best use of police resources under different scenarios.
The micro-modelling approach is helping the cities of Manchester and Sheffield, along with NHS Scotland, to examine the long-term effects of policies on public health through the UK Research and Innovation (UKRI) and charity-funded SIPHER project. The focus is on which early years policy interventions will have the most long-term benefit in the complex and interrelated areas of housing, economic growth, adverse childhood experiences and mental health.
Many city agencies from local NHS trusts to transport planners and local councils, depend on reliable data to forward plan, but often important data sets contain highly confidential personal information which limits their use. Alison and colleagues, through the QIPP project, are using their agent-based modelling expertise to create highly accurate simulated datasets from health and business data, which protect privacy and identity and yet make individual level data more widely available for a wide range of planning purposes.
Understanding uncertainty and developing new modelling methods
A problem for modellers is the many uncertainties buried in the data and assumptions required to build the individual scale models. Through Alison’s Economic and Social Research Council (ESRC) Fellowship with the Alan Turing Institute, she is working to develop methods that recognise and reduce the uncertainty which will produce more accurate predictions in future.
Understanding the hidden patterns and processes within the data is also a key part of Alison’s work, and the team are also adapting causal inference modelling, a method used in epidemiology, to better understand causal relationships in urban population data. This could help to answer key questions for city planning such as what could happen if an area is pedestrianised – will it cause more people to take active travel and how might that affect obesity prevalence?
“Taking methods from causal inference modelling, adapting those to the sort of data in urban analytics and feeding this into agent-based models can improve calibration and validation of the agent-based models” explained Alison.
Alison’s team are also using the university’s interdisciplinary culture through the Alan Turing Institute and the Leeds Institute for Data Analytics (LIDA), to work with mathematicians, physicists and engineers to adapt their probabilistic models for use with urban data.
Contact us
If you would like to discuss this area of research in more detail, please contact Professor Alison Heppenstall.